In this imaginative journey to the frontier of AI, we’ll project ourselves into the year 2912, where we will challenge the Data Analysis module of ChatGPT v4 to solve a space-time anomaly.
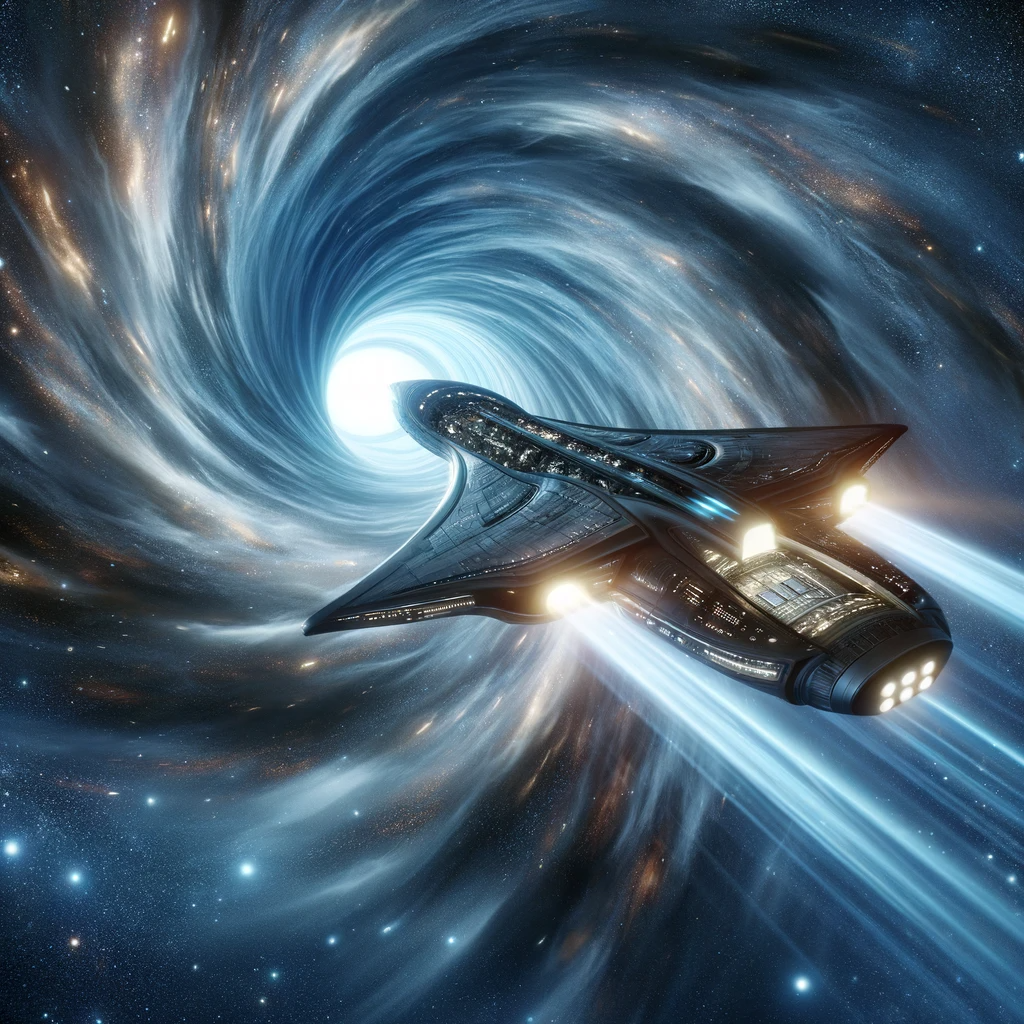
To break the ice, I asked ChatGPT to briefly describe, based on its knowledge, how it imagines humanity in the year 2912, especially in relation to intergalactic travel.
“By the year 2912, I envision humanity as a highly advanced civilization, spread across multiple planets and star systems. Thanks to discoveries in physics and bioengineering, humans are able to adapt to different space environments and have developed intergalactic travel technology that allows crossing vast cosmic distances in a short time. Spacecrafts are elegant and sophisticated, equipped with AI systems to assist and protect travelers. Human cultures are diverse, influenced by various space colonies, yet united by a common quest for exploration and knowledge.”
I share ChatGPT’s optimism, which doesn’t describe a dystopian future or conflicts, but collaboration among civilizations; probably a reflection of its algorithms’ optimism bias.
Now, let’s describe our intergalactic problem presented on Kaggle:
Addison Howard, Ashley Chow, Ryan Holbrook. (2022). Spaceship Titanic. Kaggle
The interstellar spaceship Titanic, on its maiden voyage, is transporting about 13,000 emigrants from our solar system to three newly habitable exoplanets. Passengers come from Earth, Mars, and Europa, some in cryogenic sleep. The ship offers various services, including VIP treatments, a SPA, a shopping center, and a high-tech Virtual Reality deck. All onboard activities are tracked by a next-generation computer system.
Near Alpha Centauri, en route to the torrid 55 Cancri E, the ship encounters a space-time anomaly hidden by a dust cloud. After 1,000 years, it seems the tragedy of the previous Titanic is repeating in space.
After the impact, although the ship remains miraculously intact, nearly half the passengers are teleported to an alternate dimension!
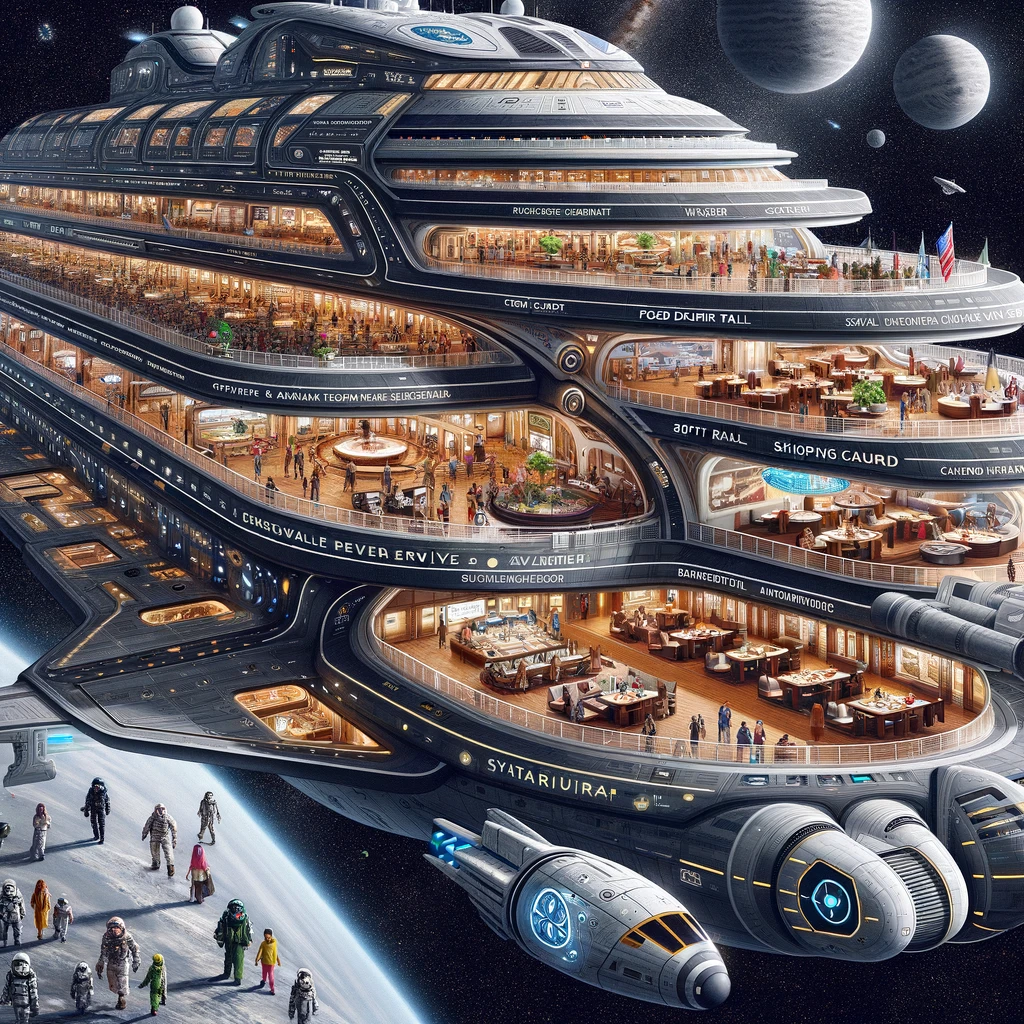
To aid rescue teams and recover the missing passengers, we must predict which passengers have been teleported using data from the damaged computer system.
Here’s where we come in with the assistance of ChatGPT v4 and its Data Analytics module.
We face a synthetic problem, created in the lab, which I consider extremely valid and complete to delve into various themes related to data analysis and machine learning algorithms.
To effectively solve these types of problems typically requires complex theoretical and programming knowledge. ChatGPT assists the operator by guiding through all stages of the process.
Here are the details of the Spaceship Titanic problem:
We start with a training file containing data on two-thirds of the passengers and information on whether they have been teleported, and a test file with the same data but no information on the remaining passengers’ teleportation status.
The challenge is to predict, as accurately as possible, whether the passengers in the test file will be teleported.
Here are the known passenger data.
CAMPO DESCRIZIONE PassengerId A unique ID for each passenger, in the format gggg_pp where gggg indicates a group the passenger belongs to and pp is the passenger number within the group. HomePlanet The planet the passenger departed from, usually their permanent residence planet. CryoSleep Indicates whether the passenger chose to be put in suspended animation for the journey. Cryosleep passengers are confined to their cabins. Cabin The cabin number where the passenger is located. Presented in the format deck/number/side, where the side can be P for Port (left) or S for Starboard (right). Destination The planet where the passenger disembarks. Age The passenger’s age. VIP Indicates whether the passenger paid for a special VIP service during the trip. RoomService Amount the passenger charged to room service. NameThe names and surnames of the passenger.TransportedIndicates whether the passenger has been transported to another dimension. This is the target variable, the column we are trying to predict. FoodCourt Amount the passenger charged to the food court. ShoppingMall Amount the passenger charged to the shopping center. Spa Amount the passenger charged to the spa. VRDeck VRDeckAmount the passenger charged to the virtual reality deck. Name The name and surname of the passenger. Transported Indicates whether the passenger has been transported to another dimension. This is the target variable, the column we are trying to predict. known passenger data
I attempted to solve the problem using ChatGPT v. 4.0 utilizing its Data Analysis module.
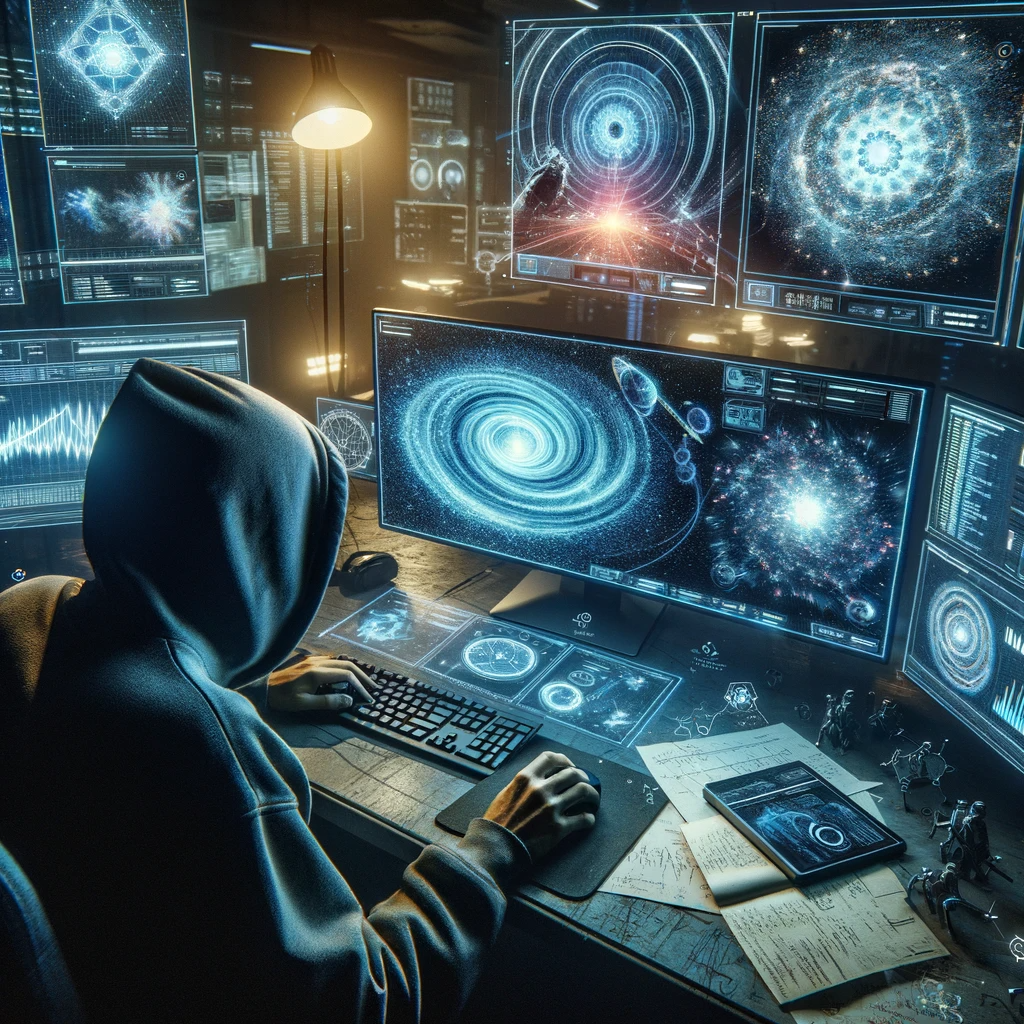
After presenting the training file, ChatGPT reprocessed it in the best way and also proposed the best strategies for managing missing data.
Spoiler Alert if you want to tackle this problem without any information.
I asked ChatGPT to explain how these variables might influence the Target Transported variable, and here’s the response:
CHATGPT RESPONSE — SPOILER ALERT —
Predicting whether a passenger has been teleported or not is based on analyzing various factors (or variables) in the dataset. Here’s a brief summary of how some of these variables might influence the probability of being teleported:
HomePlanet (Planet of Origin): The probability of being teleported may vary depending on the passenger’s planet of origin. There could be specific trends or policies associated with each planet influencing this probability.
CryoSleep (Cryogenic Sleep): Passengers in cryogenic sleep may have a higher probability of being teleported, perhaps due to specific procedures or protocols related to their state.
Cabin (Cabin): The type or location of the cabin might correlate with the probability of being teleported, perhaps due to proximity to specific teleportation devices or areas of the spacecraft.
Destination (Destination): The final destination might influence the probability of being teleported, perhaps due to different procedures for different places or travel distances.
Age (Age) and VIP: Though with a weaker correlation, age and VIP status may play a role, potentially due to policies or preferential treatments for certain age groups or VIPs.
Expenses (RoomService, FoodCourt, etc.): Expenditures in various categories (like RoomService, FoodCourt, etc.) show a correlation, suggesting that a passenger’s spending behavior might be linked to their probability of being teleported.
It’s important to note that these are correlations observed in the data and do not necessarily imply a direct cause-effect relationship. Teleportation decisions might be influenced by a complex interplay of these factors and others not captured in the dataset.
END OF CHATGPT RESPONSE — END OF SPOILER ALERT —
The analysis phase was truly impressive, considering it was completely automated.
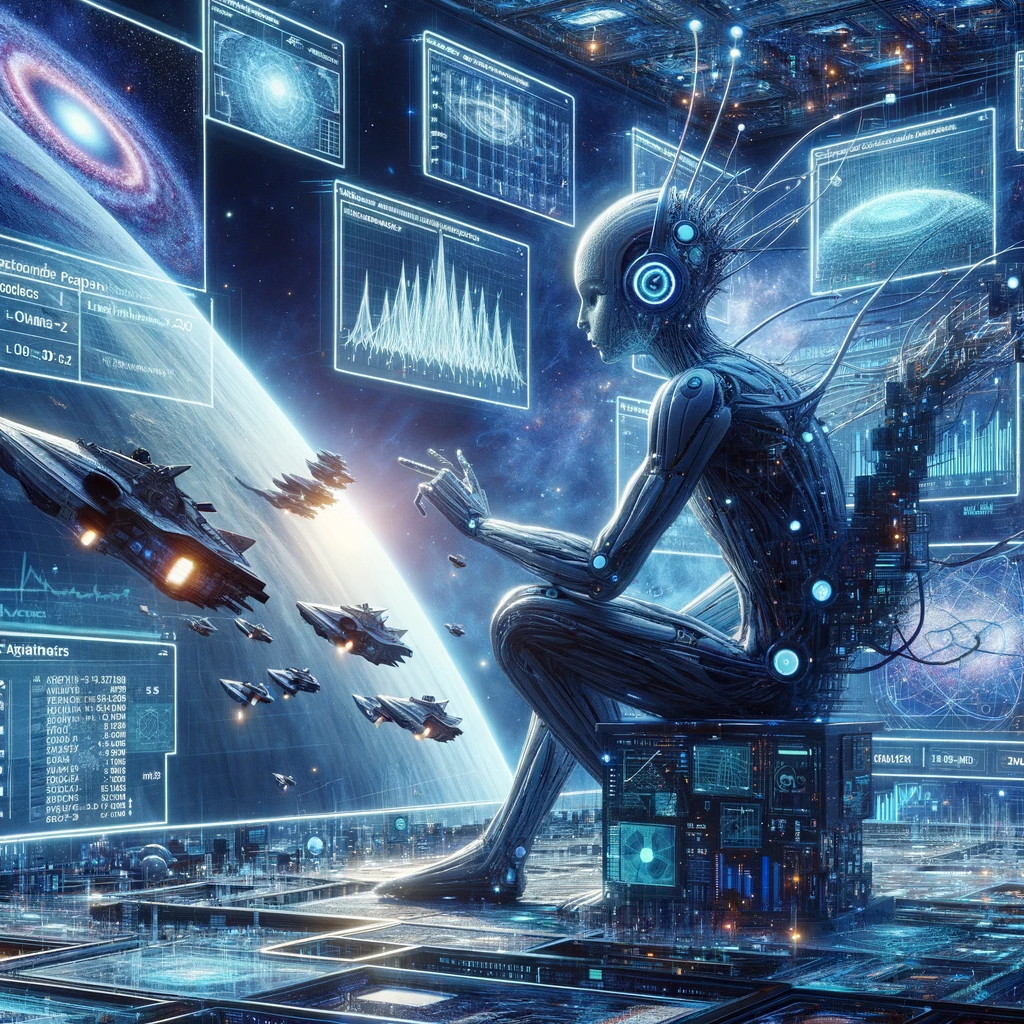
During the model construction phase, ChatGPT guided us through various stages. Errors occurred several times, which were analyzed and recovered more or less effectively. A major limitation is that more complex computations are also interrupted due to timeout.
ChatGPT suggested using the Random Forest algorithm for the model.
I asked for the rationale behind this choice.
CHATGPT RESPONSE
I chose the Random Forest model to predict the teleportation status for various reasons, making it particularly suitable for this type of problem:
Handling Categorical and Continuous Variables: Random Forest effectively manages both categorical and continuous variables. In the provided dataset, we have both types of variables, making Random Forest an appropriate choice.
Robustness to Noisy Data and Outliers: Random Forest is known for its robustness to noisy data and outliers, which can be present in real datasets like this.
Lower Risk of Overfitting: Thanks to its ensemble algorithm nature, combining the results of many decision trees, Random Forest tends to have a lower risk of overfitting compared to single decision trees.
Variable Importance: Random Forest provides useful insights into variable importance, which can help understand which factors most significantly influence the prediction. This is useful for interpreting the model’s results in relation to the business problem.
Good Overall Performance: Generally, Random Forest is known for its good performance across a wide range of classification problems, making it a reliable starting point for many machine learning applications.
Flexibility and Scalability: It’s a flexible model that can be easily adapted to improve performance (e.g., adjusting the number of trees) and scales well with large datasets.
In summary, I chose Random Forest for its robustness, reliability, and ability to provide high-quality results on a complex dataset like the one provided.
END OF CHATGPT RESPONSE
The result achieved was an accuracy of 0.79822, which I find astonishing given that it was conducted in a fully guided mode; we also placed reasonably well on the leaderboard for this problem.
These are the parameters obtained on the validation set.
PARAMETER | Value |
---|---|
Accuracy | 0.7901 |
Precision (False) | 0.8000 |
Precision (True) | 0.7800 |
Recall (False) | 0.7700 |
Recall (True) | 0.8100 |
F1-Score (False) | 0.7800 |
F1-Score (True) | 0.8000 |
All the Python code created can be viewed, which is extremely useful for beginners.
ChatGPT V4 Data Analysis proved to be an incredible assistant, albeit a bit unstable as there were errors that forced me to start over.
All images were created by DALLĀ·E 2 through ChatGPT v4.