Imagine sitting on your canary-yellow couch, sipping an ice-cold Duff with Homer, as Bart throws out a few quips and Lisa tries to correct you on every topic. Now, picture this: your one and only mission is to build a convolutional neural network (CNN) that can accurately recognize each member of the Simpsons cartoon. Because, with all that yellow around, even the best deep learning models might slip up!
The mission is clear: identify Homer, Bart, Marge, and the entire cast of Springfield with high precision and robust performance, as the images are dynamic, and the characters aren’t in obvious poses or positions.
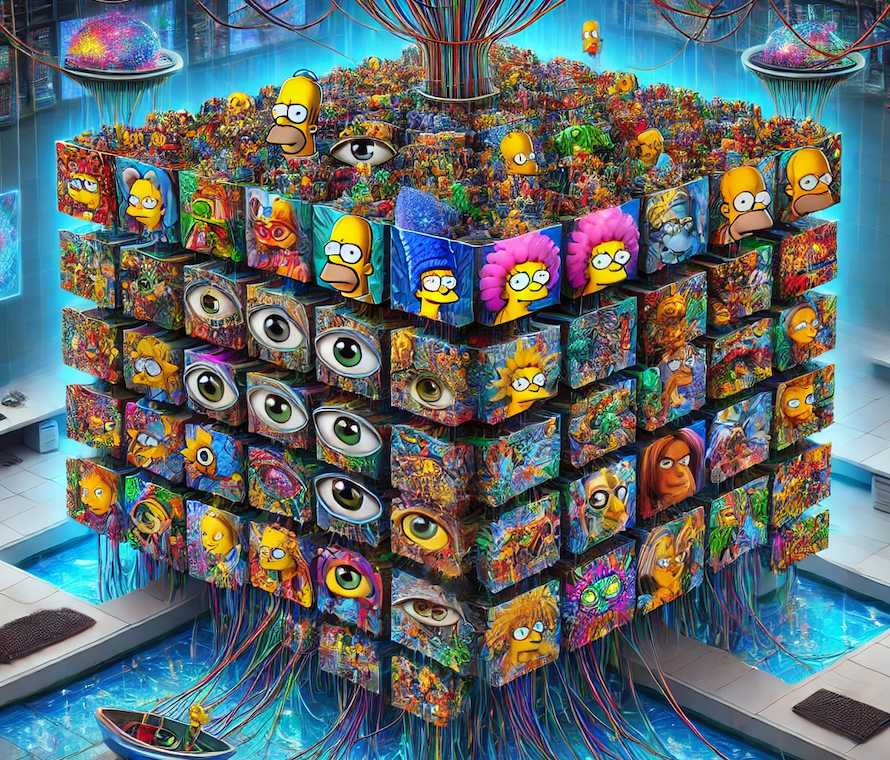
Let’s kick things off with a spoiler: the ConvNet we built achieved extraordinary results on every front.
The outstanding accuracy achieved in recognizing characters on any new image (not used during training) is: 93.65%
ANALYZE, REVIEW, AND FREELY MODIFY THE KAGGLE NOTEBOOK WITH THE SOURCES HERE
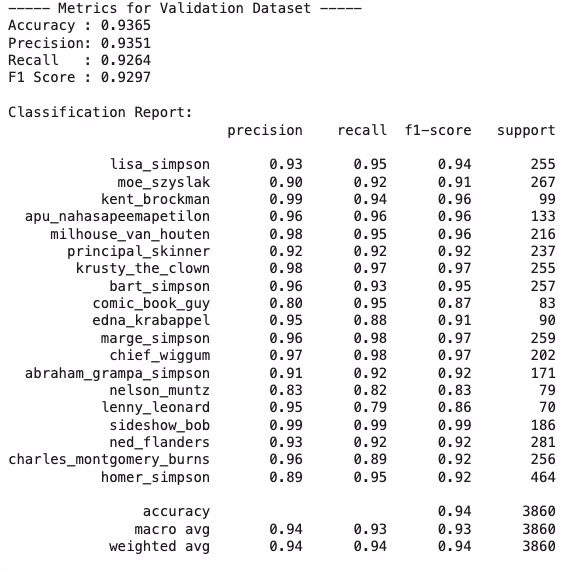
How did I achieve this? I built my best CNN, trained using transfer learning and enhanced with data augmentation to improve robustness and generalization. This challenge was a magical doorway, plunging me once again into the fascinating world of deep learning—a parallel dimension where every pixel offers a new opportunity to experiment with and dive into cutting-edge techniques. And so, once again, after long workdays, I found myself on the metro, in the car, or even in some magical corner, studying and coding.
The dataset I ventured into is the famous “The Simpsons Characters Data” (by Alexandre Attia) available on Kaggle: 16,670 images covering a whopping 43 different characters from Springfield. After acquiring it, I stumbled upon the first challenge—worthy of a Simpsons-style slip on a banana peel: the dataset is highly imbalanced. Main characters have thousands of images (Homer, Bart, Lisa), while others have only a few (poor Lionel Hutz and Disco Stu!).
To balance the dataset, I had to set a threshold: only characters with at least 200 images were included in the training. This allowed me to focus on well-represented characters. With this selection of Springfield’s finest, the CNN had a solid foundation to start accurately recognizing the most iconic characters.
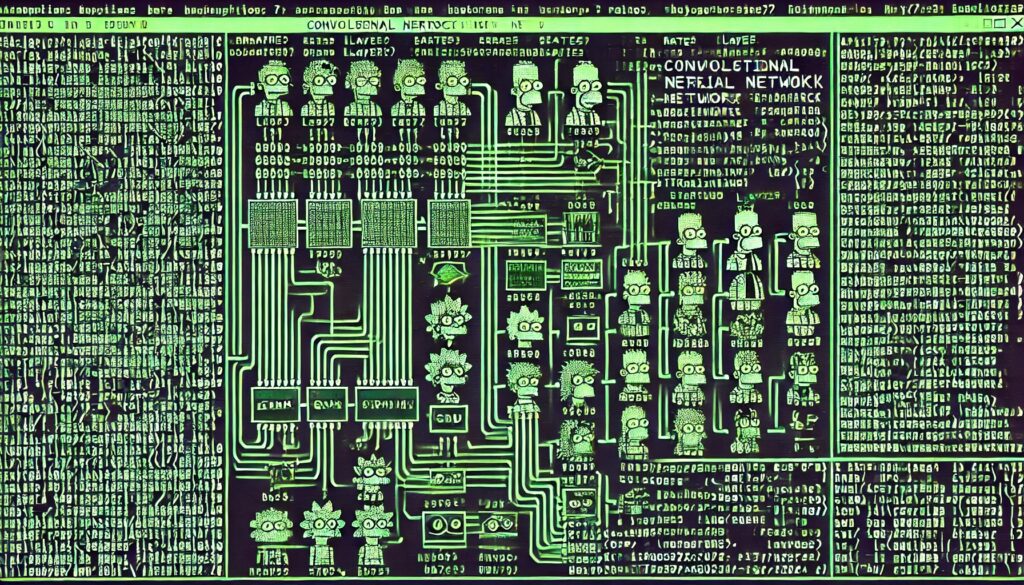
Transfer learning is a technique where you take a pre-trained model (such as one trained on ImageNet) and “transfer” its knowledge to solve a specific new task, like recognizing the Simpsons characters. This approach allows you to leverage the initial layers, already ‘skilled’ at recognizing general patterns (shapes, colors, textures), so you can focus on fine-tuning only the final layers to distinguish the unique details of each character. Generally, for this type of problem, it’s an excellent solution.
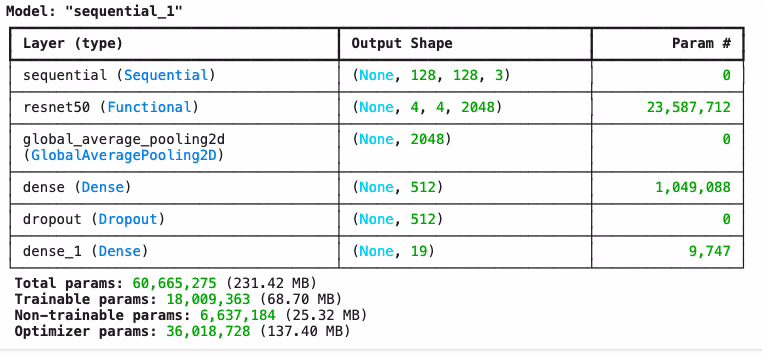
Identifying the overall structure is an art akin to magic. For this project, I sought a blend of flexibility and robustness: data augmentation enhances generalization, the base model leverages the power of transfer learning, and GlobalAveragePooling2D reduces parameters while retaining essential information. The dense layer with 512 neurons captures the main features, while Dropout prevents overfitting and boosts robustness, making the model less reliant on individual neurons and more capable of generalizing to new data. The softmax output enables accurate classification among the various characters.
Choosing ConvNet50 as the pre-trained base model felt like selecting the best superhero to tackle the battle for Simpsons recognition! Also known as ResNet50, this network is built on a 50-layer deep structure designed to scrutinize every pixel with microscopic precision. ConvNet50 is pre-trained on ImageNet, a dataset that has processed millions of images and learned to recognize even the smallest details. This means that, even if it’s never seen Homer, Bart, or any Simpsons episode, it already knows how to identify shapes, colors, and textures to near perfection.
Why ConvNet50? First, this network’s design incorporates an architecture based on residual blocks. These blocks help maintain the information flow even in a deep network like this one, ensuring high accuracy and reducing the risk of vanishing gradients. It’s like giving the network the best GPS possible, so it always knows where it’s heading, no matter how long the journey. In our case, this means a network that stays on track, avoiding drift into errors and maintaining focus even on the finest details.
Another base model alternatives I explored:
- InceptionV3: Another deep learning powerhouse, known for its ability to handle multiple scales within images. InceptionV3 could have offered greater flexibility in recognizing details like Marge’s big blue hair or Bart’s rebellious spikes, as it explores different dimensions of the same image. Often oversized, InceptionV3 is ideal for scenarios with highly intricate details that require multi-scale detection.
- VGG16 or VGG19: Widely used and simpler in structure compared to ConvNet50, yet deep and precise. These networks perform excellently in many computer vision applications, though they tend to be less efficient in terms of resources.
- MobileNet: Ideal if my goal were to deploy the model on mobile or low-resource devices. MobileNet is fast and lightweight—an extreme nerd choice if I wanted my CNN to run on an embedded system. But for the Simpsons, opting for a more powerful model made sense!
Ultimately, ConvNet50 offers the perfect balance: it’s deep enough to capture the complexities of Springfield’s characters, well-trained to capture even the smallest details, and optimized to provide fast and efficient results without overloading the system.
To achieve excellent results, I incorporated data augmentation, an essential technique for improving model performance, especially when the dataset is imbalanced or limited in the number of images. In our case, data augmentation allows us to virtually increase the size of the dataset by creating variations of existing images (through rotation, zoom, contrast, and horizontal reflection).
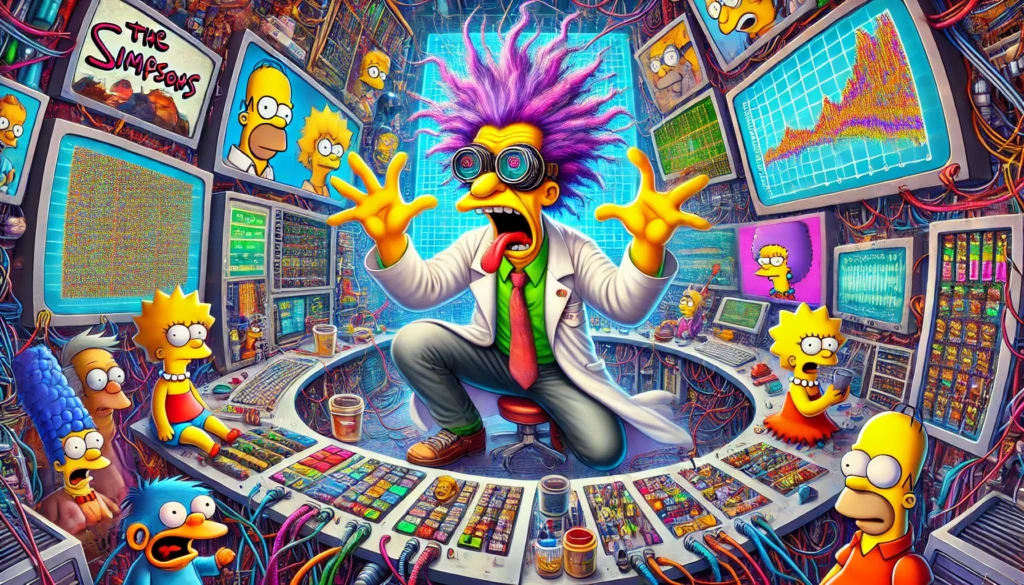
After transfer learning, where we unlock and retrain only the final layers of the pre-trained model, I applied fine-tuning, unlocking and retraining only the last layers of the model. This approach allows the model to specialize in the details of the Simpsons characters, retaining general knowledge while adapting to the specific nuances of the new dataset.
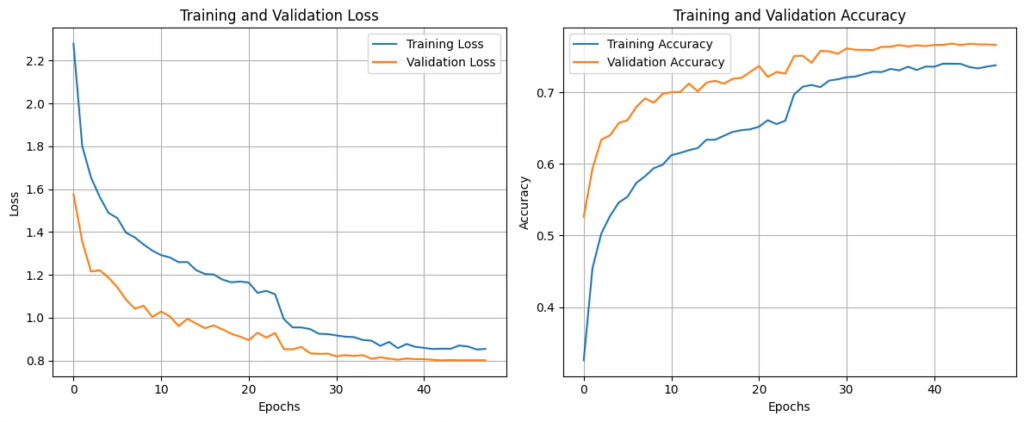
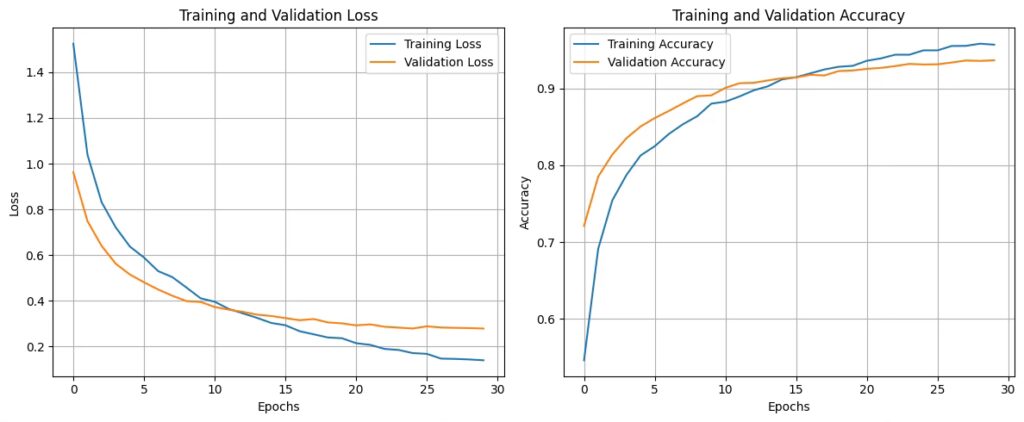
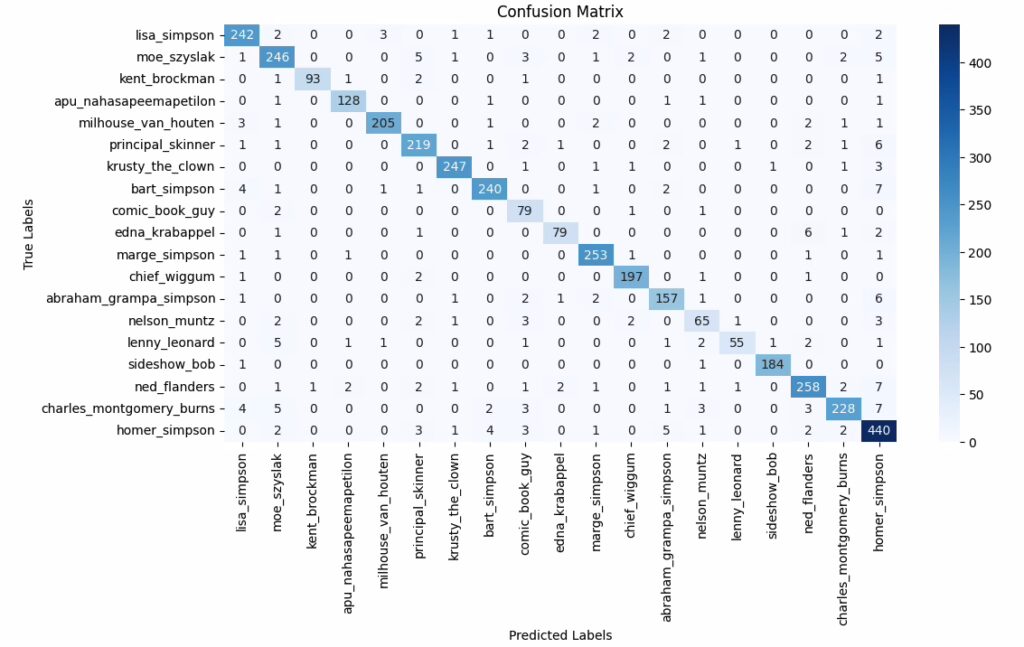
We present the simulation on images from the dataset not used in training, showcasing the actual character, the recognized character, and the network’s confidence level. These are images the network has never seen before, yet it identifies them with a high degree of certainty. Click on the images to view them in detail.
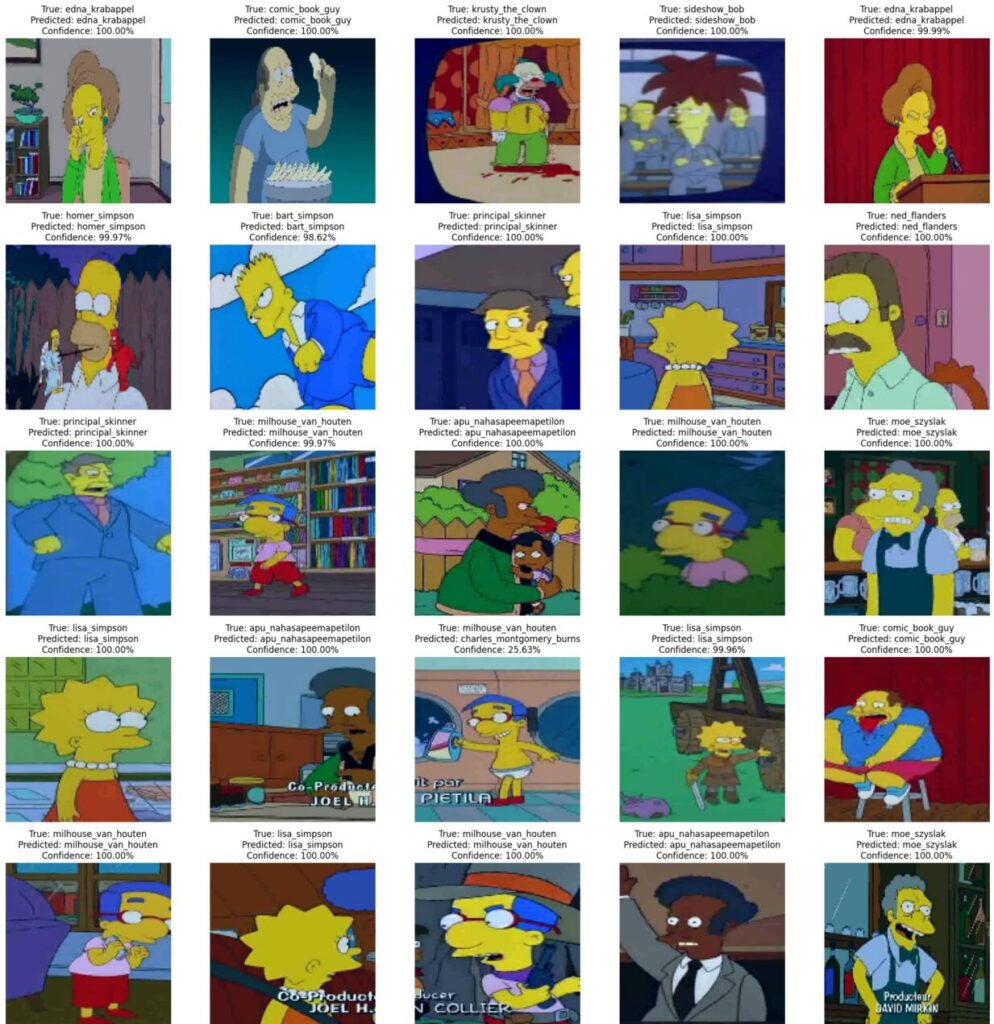
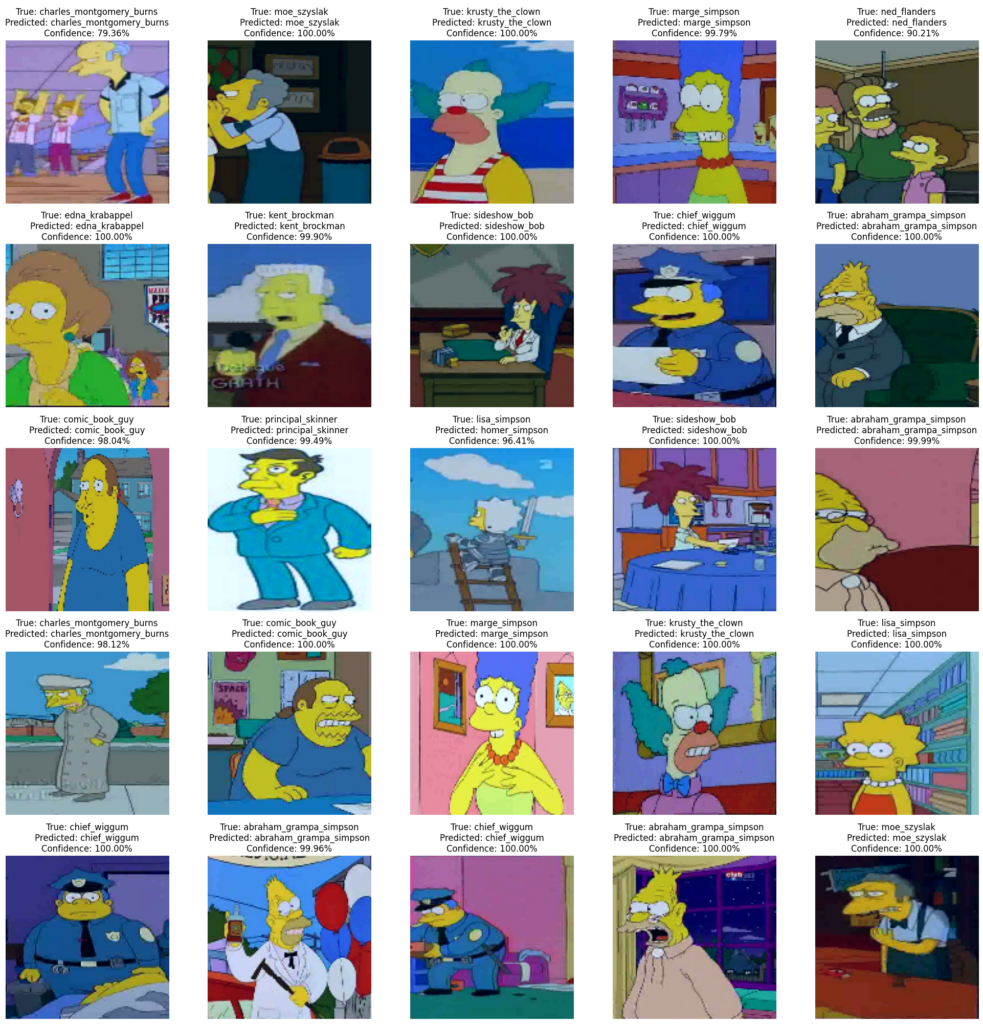
ANALYZE, REVIEW, AND FREELY MODIFY THE KAGGLE NOTEBOOK WITH THE SOURCES HERE
This project is highly reusable for numerous similar applications. Depending on the project’s requirements, one could adjust the model’s structure or choose a different base model.
“In the wise words of Homer Simpson, ‘Mmm… data!’ With this model, even Springfield would be proud of our machine learning journey!”
The charts and model performance metrics are based on a single execution of the notebook. With each run, these values may vary slightly, yet they consistently remain optimal and align closely with the results shown.
If this topic has sparked your interest and you’d like to delve deeper, I suggest these references:
Kaggle – Convolutional Neural Network (CNN) Tutorial – Kaggle
Coursera – Convolutional Neural Networks by Andrew Ng
Stanford University – CS231n: Convolutional Neural Networks for Visual Recognition